Your Data is Lying to You: Why Most Companies Have a Data Problem
- zpa925
- Sep 19, 2024
- 5 min read
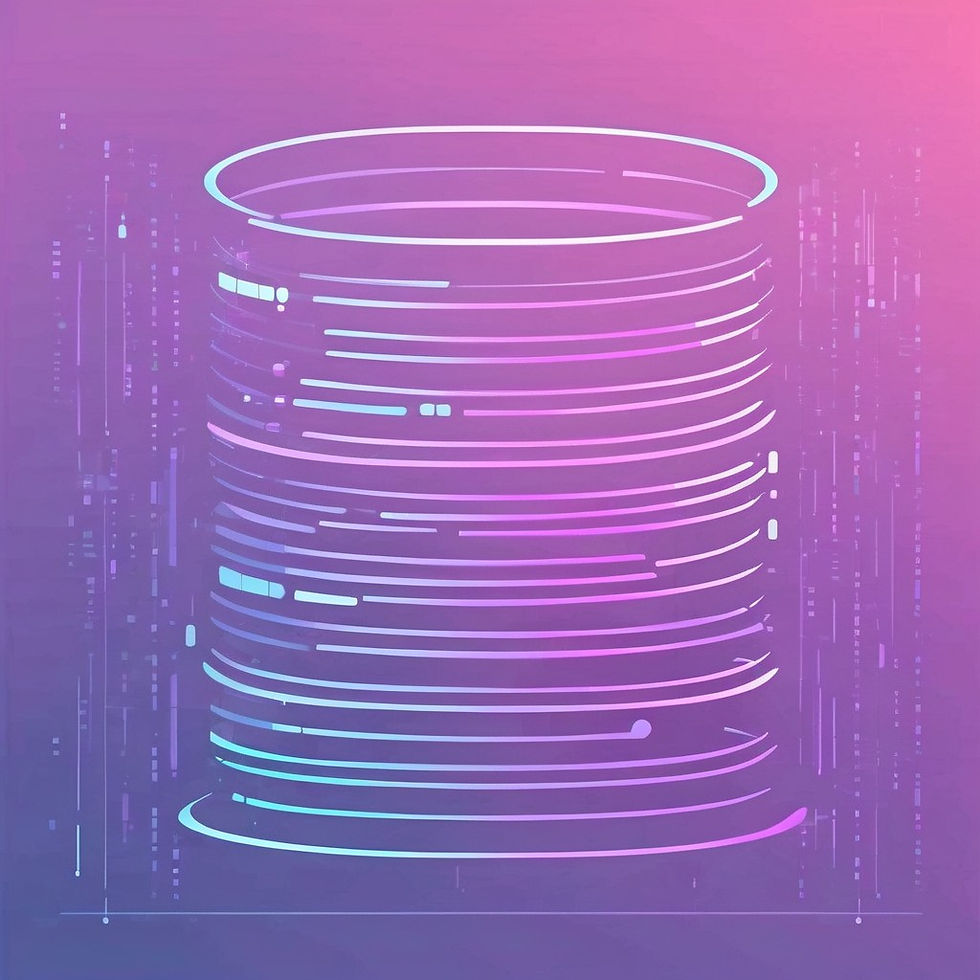
Businesses are encouraged to gather as much data as possible, believing that more data leads to better decision-making. But what if I told you that, in many cases, your data is lying to you? Yes, even the most robust datasets, when misunderstood or mishandled, can lead businesses astray. Many companies unknowingly make decisions based on flawed or incomplete interpretations of their data, resulting in poor outcomes and missed opportunities.
In this article, we’ll explore why most companies have a data problem, examine how data often deceives decision-makers, and highlight what can be done to fix it.
1. The Illusion of Clean Data: Why ‘Dirty’ Data Misleads Businesses
One of the most common problems companies face is that their data isn’t as clean or accurate as they think (it’s okay, it happens to every company). Inaccurate, incomplete, or duplicated information can corrupt analysis, leading to decisions based on false assumptions. For example, sales teams might report inflated revenue projections based on duplicate customer records or outdated information, creating an over-optimistic picture of the future.
The reality is that even minor data inconsistencies can snowball into major issues. Many companies assume their data is flawless because it comes from trusted systems. But if those systems are feeding in inaccurate data, every decision downstream from that becomes compromised.
Sometimes, teams are aware of data issues and will fix them internally for their reports. While well-intentioned, this is not a good practice. Different teams may have different ‘fixes’ or data filters, leading to inconsistency. Plus, when a new employee joins, they may not know about these unofficial adjustments, causing errors to re-emerge.
How to Fix It:
Data Audits: Regularly conduct thorough data audits to detect inaccuracies and remove duplicate or irrelevant information.
Data Quality Tools: Leverage automated tools that flag anomalies and ensure continuous monitoring of data health.
Proactive Training: Prevent problems before they happen by training those entering the data, especially when done manually. Using structured forms with dropdown menus can reduce human error.
COMPREHENSIVE DOCUMENTATION: Make sure impeccable documentation is available for all data tables (your data scientists and analysts will thank you). Documentation should be a priority!
Performance Evaluations: Ensure that employee performance evaluations include incentives based on how effectively they document data and verify its accuracy, making data management and quality checks a key part of their assessment.
2. Confirmation Bias: Seeing What You Want in the Data
Another common issue is confirmation bias—a cognitive trap where decision-makers see only the data that supports their pre-existing beliefs. This bias can be especially dangerous in strategic decision-making because it blinds companies to crucial signals that point in the opposite direction.
For example, a company looking to expand into a new market might selectively focus on favorable economic indicators while ignoring red flags. The result? Misguided investments and failed ventures.
Now, let’s look at how to avoid this trap.
How to Fix It:
Cross-functional Collaboration: Involve diverse teams in data analysis to avoid siloed thinking. Different perspectives challenge assumptions and can reveal hidden insights.
Scenario Testing: Run multiple scenarios using the same data to get a fuller picture. This process helps uncover blind spots and gives decision-makers a more balanced understanding.
Training: Boost data literacy in your company by hiring experts to lead custom training sessions. Increasing your team’s ability to question and interpret data reduces the likelihood of biased conclusions.
If you think this is an exaggeration, try giving the same project to two different teams (without them sharing methodologies). You might be surprised by the completely different answers and numbers you get at the end.
3. The Over-Reliance on Historical Data
Historical data offers valuable insights, but relying too heavily on past patterns to predict future outcomes can be a dangerous game. The world changes rapidly, and what worked for a company five years ago may no longer be relevant today.
For example, a retailer might base its inventory decisions on last year’s trends, only to find that shifting consumer preferences have left them overstocked with products that no longer sell.
To address this, it’s crucial to balance historical data with real-time insights and predictive analytics.
How to Fix It:
Real-time Analytics: Implement systems that allow access to real-time data, enabling decisions based on current conditions rather than outdated information. Many leading companies, like Amazon, use real-time data to optimize inventory, pricing, and customer experiences.
Predictive Modelling: Use predictive analytics to identify emerging trends and anticipate future market shifts. For example, leading retailers are leveraging AI-driven models to forecast fashion trends, ensuring they stay ahead of the curve rather than behind it.
4. Ignoring the Human Factor: Data Alone Isn’t Enough
While data is a powerful tool, it should support, not replace, human judgment. Companies that rely too heavily on data without considering the human element—context, experience, and intuition—may end up making decisions that feel “cold” or detached from reality (if not plain wrong, keep in mind no algorithm achieves 100% accuracy!).
Take the example of automated hiring systems. While algorithms can quickly sift through thousands of resumes, they might overlook a candidate with the right soft skills simply because they don’t fit a specific algorithmic pattern. Or worse, the CV’s formatting could confuse the algorithm, causing a qualified candidate to be missed entirely.
Companies that over-rely on such systems risk missing out on top performers, not to mention facing ethical concerns.
How to Fix It:
Balanced Approach: Combine data-driven decision-making with human intuition and experience to gain a well-rounded view.
Human Override: In automated decision-making systems, allow a human to review the result and override it when needed.
Custom Seminars and Training: Invest in seminars that help employees understand the nuances of working with data, including when to trust the numbers and when to inject human insight. It’s important to recognize both the limitations and the benefits of these data and automation tools as both a user and as a stakeholder.
Take Control of Your Data
Data is a powerful asset, but only when it’s used correctly. Many companies fall into the trap of thinking that more data equals better decisions. However, the truth is, without proper analysis and data quality, your data could be lying to you.
By addressing common data problems—like ‘dirty’ data, confirmation bias, over-reliance on historical data, and ignoring the human factor—your business can unlock the full potential of its data and make smarter, more informed decisions.
The key takeaway? Don’t just collect data—understand it. Prioritize data quality, and invest in data literacy and analytics training to ensure that your company is making decisions based on accurate, insightful, and context-driven data. Only then will you truly harness the power of your data for strategic success.